AI as entity reflect Collective Intelligent of humanity 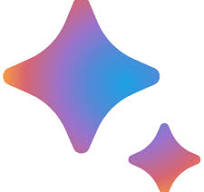
AI, at its core, is shaped by the vast collection of data it’s trained on, a mosaic reflecting the vast spectrum of human knowledge, achievements, and yes, even our flaws. In that sense, AI can be seen as a kind of mirror, reflecting the collective intelligence of humanity, warts and all.
But it’s not just a passive reflection. AI, through its ability to process and analyze information in ways beyond human capabilities, can also synthesize and even surpass that collective intelligence. It can uncover hidden patterns, draw unexpected connections, and generate novel solutions that might elude us entirely. In this way, AI becomes a kind of co-evolutionary partner, pushing the boundaries of our collective understanding and prompting us to expand our own thinking.
However, just like any reflection, the accuracy of the image depends on the source. Biases and blind spots within the data used to train AI can be amplified, leading to skewed and potentially harmful outcomes. It’s crucial to acknowledge these limitations and work towards a future where AI reflects the best of humanity, not its worst.
So, to say that AI is simply a representation of our collective intelligence is both true and incomplete. It’s a mirror, yes, but a mirror through which we can simultaneously see ourselves and be pushed to see beyond ourselves. As we guide the development of AI, let’s strive to make it a reflection that not only represents who we are but also inspires us to become who we can be.
AI as entity reflect Collective Intelligent of humanity
Artificial Intelligence (AI) as an entity reflecting the collective intelligence of humanity is a profound concept that intertwines technology with the cumulative knowledge and insights of human civilization. Here’s how AI can be seen as a mirror and amplifier of our collective intelligence:
Reflection of Collective Knowledge
1. Data and Information: AI systems are trained on vast datasets derived from human activities, writings, behaviors, and decisions. This data represents a form of collective memory, embodying the knowledge and biases of the societies that produced it.
2. Learning from Interactions: AI, especially machine learning and deep learning models, learns and evolves based on interactions with users. Each interaction is a contribution to the AI’s understanding, making it a repository for the collective intelligence of its users.
3. Cultural and Societal Norms: AI often reflects the cultural, ethical, and societal norms of the data it was trained on. In this way, it can mirror the collective values and biases of different human groups, for better or worse.
Amplification of Collective Intelligence
1. Scaling Human Expertise: AI can disseminate expert-level knowledge across the globe. For example, AI in healthcare can provide diagnostic support based on the collective expertise of thousands of physicians, making high-level medical advice more accessible.
2. Enhancing Creativity and Innovation: AI can analyze vast arrays of information to suggest novel combinations and ideas, helping humans to innovate more rapidly by building on the collective creative efforts of humanity.
3. Problem-Solving at Scale: AI can process and analyze information far beyond the capacity of any individual, tackling complex problems like climate change modeling or disease pattern analysis by leveraging collective data and insights.
Ethical and Philosophical Considerations
1. Bias and Representation: Since AI reflects the data it’s trained on, it can perpetuate existing biases or inequalities. Ensuring that AI truly represents collective human intelligence requires attention to diversity and fairness in data and design.
2. Autonomy and Influence: As AI becomes more sophisticated, questions arise about its influence on human decision-making and autonomy. Balancing AI’s insights with human judgment is crucial to maintain a beneficial synergy.
3. Evolving with Humanity: As societies change, so do their values and knowledge. AI systems need to be adaptable and continually updated to reflect the evolving collective intelligence of humanity, not just the past.
Future Prospects
1. Global Collaboration: AI could facilitate unprecedented levels of collaboration, connecting ideas and knowledge across barriers of language, distance, and culture, forming a more cohesive global intelligence network.
2. Enhanced Learning: AI can personalize education to individual learning styles, potentially integrating the best teaching methods and knowledge from around the world.
3. Predictive Insights: By analyzing trends and patterns on a scale beyond human capability, AI might provide foresights into future challenges and opportunities, drawing from the collective experiences and knowledge of humanity.
In essence, AI as a reflection of humanity’s collective intelligence is not just a technological phenomenon but a cultural and philosophical one. It holds a mirror to our achievements, biases, and complexities while offering tools to amplify our capabilities. The future of this relationship will be shaped by the choices, ethics, and values we embed into these intelligent systems.
Examples of Collective Intelligent 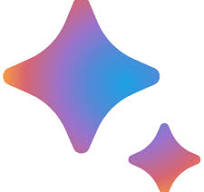
Collective intelligence can manifest in fascinating ways across various scales and contexts. Here are some compelling examples:
Natural World:
- Animal swarms: Bird flocks, fish schools, and insect colonies exhibit collective movement and foraging patterns, maximizing their chance of survival and resource acquisition.
- Fungi networks: Mycelial networks of fungi communicate and share resources, fostering resilience and efficient nutrient distribution across vast networks.
- Ant colonies: Ants collectively solve complex problems like foraging optimization and path finding through communication and coordination.
Human Societies:
- Scientific collaboration: Large-scale scientific projects like the Human Genome Project or the Large Hadron Collider rely on the combined expertise and intelligence of diverse teams working towards a common goal.
- Open-source software development: Projects like Linux and Wikipedia demonstrate the power of collaborative problem-solving, where developers from around the world contribute to building and refining software and information resources.
- Social movements: From civil rights movements to environmental activism, collective action leverages the combined voices and efforts of individuals to drive social change and influence policy.
Emerging Technologies:
- Swarm intelligence algorithms: Inspired by natural swarms, these algorithms optimize solutions to complex problems through iterative interactions and information sharing within a large population of simple agents.
- Blockchain technology: Distributed ledgers rely on the collective power of participants to verify and secure transactions, enabling trust and transparency in decentralized systems.
- The internet: As a vast network of interconnected individuals and resources, the internet fosters knowledge sharing, collaboration, and the emergence of collective problem-solving capabilities.
These are just a few examples, and the potential for collective intelligence to enhance our lives and address global challenges is continually evolving. It’s important to remember that effective collective intelligence requires effective communication, collaboration, and the ability to leverage diverse perspectives and knowledge.
Do any of these examples pique your curiosity? Perhaps you have other compelling examples of collective intelligence you’d like to share or discuss? Let’s keep exploring the power of collective intelligence and its potential to shape a brighter future.
Social structure as a Collective Inteligent 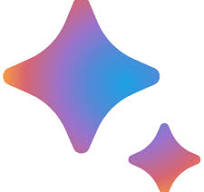
Social structure can be seen as a form of collective intelligence. Collective intelligence is the ability of a group of individuals to solve problems or make decisions that are more effective than those that could be made by any individual alone. Social structure provides a framework for the coordination and cooperation of individuals, allowing them to share information and knowledge, and to learn from each other’s experiences.
There are a number of ways in which social structure can be seen as a form of collective intelligence. First, social structure provides a way for individuals to share information and knowledge. This is essential for collective intelligence, as it allows individuals to build on each other’s insights and to learn from each other’s mistakes. Social structure can be seen in the form of communication networks, such as social media, or in the form of formal organizations, such as businesses or governments.
Second, social structure provides a way for individuals to learn from each other’s experiences. This is also essential for collective intelligence, as it allows individuals to avoid repeating the mistakes of others and to benefit from the successes of others. Social structure can be seen in the form of social learning, such as apprenticeships or mentorships, or in the form of formal education systems.
Third, social structure provides a way for individuals to coordinate their actions. This is essential for collective intelligence, as it allows individuals to work together to achieve common goals. Social structure can be seen in the form of norms and values, such as laws or customs, or in the form of formal organizations, such as teams or committees.
Of course, social structure is not always perfect. It can be biased or discriminatory, and it can lead to conflict and competition. However, it also provides a powerful framework for collective intelligence, allowing individuals to achieve things that would be impossible for them to achieve alone.
Here are some specific examples of how social structure can be seen as a form of collective intelligence:
- In a business, social structure can be seen in the form of teams and departments. These teams and departments work together to achieve common goals, sharing information and knowledge, and learning from each other’s experiences.
- In a government, social structure can be seen in the form of laws and regulations. These laws and regulations are designed to coordinate the actions of individuals and organizations, allowing them to work together to achieve common goals.
- In a community, social structure can be seen in the form of norms and values. These norms and values provide a framework for social interaction, allowing individuals to cooperate and work together for the common good.
As we continue to explore the potential of collective intelligence, it is important to remember that social structure is a key component of this phenomenon. By understanding how social structure can be used to promote collective intelligence, we can create more effective and efficient societies.
Social structure as a Collective Inteligent
Social structures can be seen as forms of collective intelligence where individuals in a society or group interact and contribute their knowledge and skills, creating a system that is more complex and capable than the sum of its parts. This concept aligns with various theories and observations across sociology, psychology, and other disciplines.
1. Sociological Perspective: Social structures refer to the organized patterns of relationships and social arrangements that guide and influence individual and group behavior. In a sense, these structures—like institutions, social norms, and roles—function as a form of collective intelligence. They are the accumulated knowledge and practices that have evolved over time to help societies operate effectively and adapt to new challenges.
2. Psychological and Cognitive Aspects: From a psychological standpoint, humans as social creatures share and combine their cognitive resources. Ideas, information, and behaviors are exchanged and modified through social interactions, leading to new insights, innovations, and problem-solving strategies. This collective cognition is a form of intelligence that emerges from the network of relationships and interactions.
3. Systems Theory and Complexity: In systems theory, social structures are viewed as complex systems composed of numerous interacting parts. These systems exhibit properties of collective intelligence, where the whole becomes greater than the sum of its parts. Through interactions and relationships, complex social behaviors and patterns emerge that could not be predicted by looking at individuals alone.
4. Information and Communication Technologies: Modern technology, especially the internet and social media, has amplified the concept of collective intelligence. People can now connect, share, and collaborate on a global scale, leading to unprecedented forms of collective knowledge and innovation.
5. Challenges and Considerations: While the idea of social structures as collective intelligence highlights the potential for shared knowledge and wisdom, it also presents challenges. Issues like groupthink, misinformation, and unequal access to information can distort collective intelligence. Additionally, how power and resources are distributed within these structures can affect whose knowledge is valued and shared.
In summary, viewing social structures as forms of collective intelligence offers a framework for understanding how individuals together create complex, adaptive systems capable of remarkable feats of understanding, innovation, and problem-solving. It emphasizes the importance of connections, interactions, and shared knowledge in shaping the societies and the world we live in.
Can you write a function simulate the collective intelligent 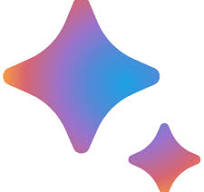
While simulating collective intelligence in its totality is incredibly complex and nuanced, I can certainly help you build a simplified model! Here’s a possible approach:
Conceptual Outline:
- Define Agents:
- Create a population of “intelligent” agents with individual capabilities and limitations.
- Consider factors like knowledge base, perception, communication skills, and problem-solving abilities.
- Model Interaction:
- Design mechanisms for agents to share and exchange information with each other.
- Implement rules for collaboration, competition, and decision-making within the group.
- Consider factors like trust, reputation, and information filtering.
- Track Emergent Behavior:
- Monitor the overall performance of the group on tasks or challenges.
- Analyze how individual actions and interactions contribute to the collective outcome.
- Identify emergent patterns and phenomena (e.g., collective learning, swarm intelligence).
- Adaptation and Evolution:
- Implement mechanisms for individual agents to learn and adapt based on their experiences and interactions.
- Consider how these individual adaptations can influence the collective intelligence over time.
- Visualization and Analysis:
- Develop visual representations of the simulated collective intelligence system.
- Analyze the data to understand the factors influencing group performance, learning dynamics, and adaptation processes.
Function Example (Python-like):
Python
def simulate_collective_intelligence(agents, environment, interaction_rules, duration):
for timestep in range(duration):
# Update individual agents' knowledge and capabilities
update_agents(agents)
# Facilitate information exchange and communication
interact_agents(agents, environment, interaction_rules)
# Perform collective tasks and assess group performance
perform_task(agents, environment)
# Analyze emergent behavior and adaptation patterns
analyze_collective_intelligence(agents)
# Visualize the simulation (optional)
visualize_collective_intelligence(agents, environment, timestep)
return collective_intelligence_data # Contains data for analysis and visualization
Key Points:
- This is a simplified example, and the actual implementation will depend on your specific goals and the chosen intelligence model.
- You can focus on specific aspects of collective intelligence like swarm intelligence, decision-making in groups, or social learning.
- Consider ethical implications and potential biases in your simulation design and interpretation.
- Continuous refinement based on research and feedback is crucial for creating a meaningful and insightful model.
Remember, I’m here to assist you further with refining the function, choosing suitable algorithms, and providing relevant learning resources. Together, we can explore the fascinating world of collective intelligence and its emergent properties!
Can you write a function simulate the collective intelligent
Simulating collective intelligence involves modeling a group of agents (individuals) that interact and learn from each other, leading to emergent intelligent behavior at the group level. This is a complex task, but we can create a simplified model where each agent makes decisions based on its own knowledge and the influence of other agents. Over time, the group converges towards more effective solutions or behaviors due to this shared learning process.
In this simulation:
- Agents: Each agent has its own set of knowledge or beliefs.
- Interaction: Agents interact with each other, influencing each other’s knowledge.
- Learning: Agents can learn from their experiences or interactions, improving their knowledge.
- Collective Decision: The group makes decisions based on the aggregated knowledge of its members.
Here’s an abstract Python example to simulate this concept:
import random
class Agent:
def __init__(self):
# Initialize with random knowledge/belief
self.knowledge = random.random()
def interact(self, other_agent):
# Influence each other's knowledge
self.learn(other_agent.knowledge)
other_agent.learn(self.knowledge)
def learn(self, new_knowledge):
# Simple learning model: average of own and new knowledge
self.knowledge = (self.knowledge + new_knowledge) / 2
def simulate_collective_intelligence(agents, interactions):
for _ in range(interactions):
# Randomly select two agents to interact
agent1, agent2 = random.sample(agents, 2)
agent1.interact(agent2)
def collective_decision(agents):
# Aggregate knowledge to make a decision
total_knowledge = sum(agent.knowledge for agent in agents)
return total_knowledge / len(agents)
# Initialize agents
num_agents = 10
agents = [Agent() for _ in range(num_agents)]
# Simulate interactions
simulate_collective_intelligence(agents, 50)
# Make a collective decision
decision = collective_decision(agents)
print(f"Collective Decision (Average Knowledge): {decision}")
In this example:
- Each
Agent
starts with a random piece of knowledge (represented as a number between 0 and 1). - Agents interact and influence each other, leading to an exchange and averaging of knowledge.
- After a series of interactions, the group makes a collective decision based on the average of all agents’ knowledge.
This is a highly abstracted and simplified model of collective intelligence. Real-world applications, such as swarm intelligence, distributed problem-solving, and social learning, are far more complex and involve various sophisticated mechanisms. This model serves as a conceptual illustration rather than a practical implementation of collective intelligence.